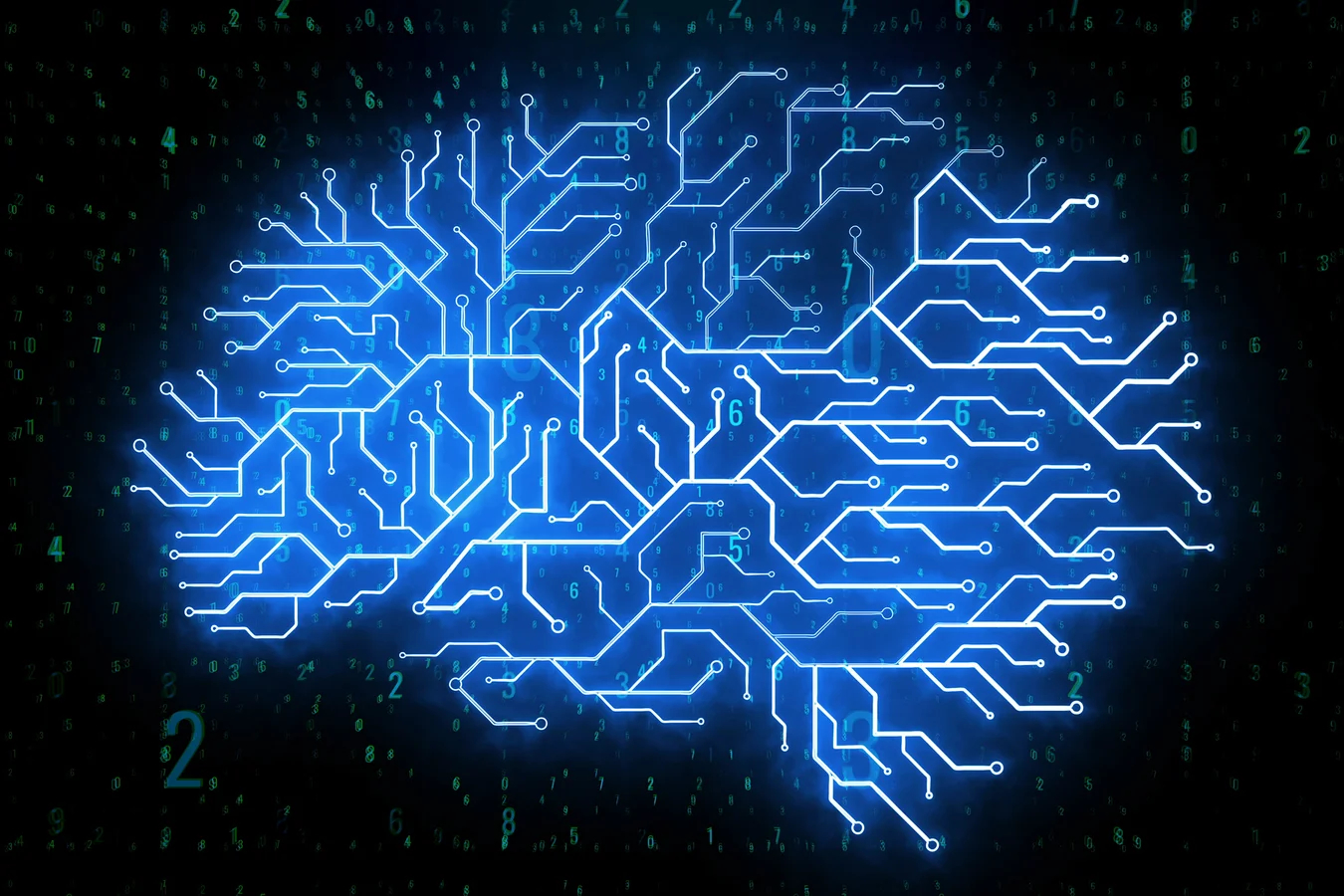
Artificial Intelligence and Machine Learning in EDI
Executive Summary
Artificial Intelligence (AI) and Machine Learning (ML) stand at the forefront of transforming Electronic Data Interchange (EDI) systems.
By automating the processing of vast amounts of transactional data, these technologies significantly reduce manual intervention, minimise errors, and enhance operational efficiency.
AI algorithms can predict supply chain disruptions, optimise logistics, and provide strategic insights, facilitating proactive decision-making. ML models learn from transaction data, improving their predictive accuracy over time.
Together, AI and ML not only streamline EDI processes but also empower businesses to adapt dynamically to market demands and supply chain challenges, paving the way for a new era of digital communication and collaboration in the business world.
Introduction
Electronic Data Interchange (EDI) systems are pivotal in digital business communications, enabling the automated exchange of standard business documents between companies.
Originating in the 1960s, EDI has evolved significantly, driven by the need to accommodate the growing complexity of global supply chains.
Modern businesses demand enhanced flexibility, scalability, and automation from EDI solutions to manage vast data volumes, ensure timely transactions, and adapt to changing market dynamics efficiently.
This evolution reflects the shift towards integrating advanced technologies to meet the intricate requirements of contemporary supply chain operations.
The Role of AI and ML in Modernising EDI
Artificial Intelligence (AI) enhances EDI systems by automating complex data processing tasks, such as order processing and invoice reconciliation, thereby reducing manual errors and improving operational efficiency.
AI’s predictive analytics capabilities enable the forecasting of supply chain disruptions before they occur, allowing businesses to take preemptive actions.
Machine Learning (ML), a subset of AI, further refines this process by analysing historical data patterns to improve the accuracy of future predictions.
This capability is crucial for optimising logistics operations, ensuring timely deliveries, and minimising costs, thus making supply chains more resilient and responsive.
Implementation Challenges
Integrating AI and ML into existing EDI frameworks presents technical challenges such as ensuring data privacy, addressing the shortage of skilled personnel, and managing significant upfront costs.
Strategies to navigate these challenges include adopting a phased implementation approach, allowing organisations to gradually integrate AI and ML technologies and assess their impact.
Investing in ongoing training and development programs is crucial to build internal expertise and reduce reliance on external vendors.
Furthermore, prioritising data security measures from the outset is essential to protect sensitive information and maintain compliance with regulatory standards.
Future Outlook
The future of AI and ML in EDI systems is likely to be influenced by the integration of Internet of Things (IoT) devices, enhancing real-time data collection and analysis for more responsive supply chains.
Blockchain could offer new levels of security and transparency, facilitating trust in automated transactions.
Advancements in Natural Language Processing (NLP) may enable more intuitive, automated communication between businesses and their EDI systems, making complex setups more accessible and efficient.
These technologies promise to further automate, secure, and streamline EDI processes, driving innovation in supply chain management.
Conclusion
Integrating AI and ML into EDI systems offers transformative potential, streamlining operations, enhancing decision-making, and predicting disruptions.
However, challenges like data privacy, skill gaps, and initial costs must be navigated.
Future advancements, including IoT, blockchain, and NLP, promise to further elevate EDI’s capabilities, making supply chains more efficient and secure.
Successfully leveraging these technologies requires strategic planning, investment in training, and phased integration, ensuring that businesses remain competitive in an increasingly digital and complex global marketplace.
References
Frontiers. (2024). Machine Learning and Artificial Intelligence.
https://www.frontiersin.org/journals/artificial-intelligence/sections/machine-learning-and-artificial-intelligence
Jair. (2024). The Journal of Artificial Intelligence Research.
https://www.jair.org/index.php/jair
Springer Nature. (2024). The Journal of Artificial Intelligence Research.
https://www.springernature.com/gp/librarians/landing/ai-machine-learning